AI Policy Advisor: Using Topic Modeling to Identify Key Issues"
- Prof.Serban Gabriel
- Aug 26, 2024
- 3 min read
Artificial Intelligence (AI) is rapidly transforming the landscape of public policy by enabling policymakers to identify and prioritize key issues based on public discourse.
One of the most effective methods for harnessing this potential is through topic modeling, a form of machine learning that analyzes large volumes of text to uncover hidden themes and trends.
This article explores how AI can be utilized to identify and prioritize policy issues, the mathematical foundations of topic modeling, and a practical example of its application.
Understanding Topic Modeling
Topic modeling is a statistical method used to uncover the latent topics present in a collection of documents.
It helps in organizing, understanding, and summarizing large datasets of textual information.
The most common algorithms for topic modeling include Latent Dirichlet Allocation (LDA) and Non-negative Matrix Factorization (NMF).
LDA assumes that documents are generated from a mixture of topics, where each topic is characterized by a distribution of words.
The key steps in LDA include initializing the model by assigning random topics to each word in the documents, followed by an iterative update process.
In this update, for each word, the topic assignment is updated based on the proportion of words in the document that belong to each topic and the proportion of words assigned to each topic across all documents.
On the other hand, NMF is another popular method for topic modeling that factorizes the document-term matrix into two lower-dimensional matrices, representing topics and their corresponding word distributions.
The objective of NMF is to minimize the difference between the original matrix and the product of the two lower-dimensional matrices, subject to non-negativity constraints.
Application of Topic Modeling in Policy Analysis
AI-driven topic modeling can significantly enhance the ability of policymakers to analyze public discourse and identify pressing issues.
By processing vast amounts of data from social media, news articles, and public comments, AI can reveal trends and sentiments that may not be immediately apparent through traditional analysis.
Example: Analyzing Public Sentiment on Climate Change Policy
A practical example of topic modeling in action is the analysis of public sentiment regarding climate change policy.
Researchers can collect a dataset of tweets, articles, and public comments related to climate change.
By applying LDA or NMF, they can identify key topics such as renewable energy, carbon emissions, and climate justice.
The process begins with data collection from various sources, followed by data preprocessing, which includes cleaning and preparing the text data.
After that, the modeling phase applies LDA to discover topics within the dataset, leading to the interpretation of the identified topics to gauge public sentiment and concerns.
For instance, if the model reveals a significant number of discussions around renewable energy and job creation, policymakers can prioritize initiatives that promote green jobs while addressing climate change.
Mathematical Explanation of Topic Modeling's Impact
The effectiveness of topic modeling in identifying key issues can be quantitatively assessed through metrics such as coherence score and perplexity.
The coherence score measures the degree of semantic similarity between high-scoring words in a topic, with a higher score indicating a well-defined topic.
Perplexity, on the other hand, is a measure of how well a probability distribution predicts a sample, with lower values indicating a better fit of the model to the data.
Challenges and Ethical Considerations
While AI and topic modeling offer powerful tools for policy analysis, there are several challenges and ethical considerations to address.
Bias in data is a significant concern, as the datasets used for analysis may reflect societal biases, leading to skewed results.
It is crucial to ensure diverse and representative data sources.
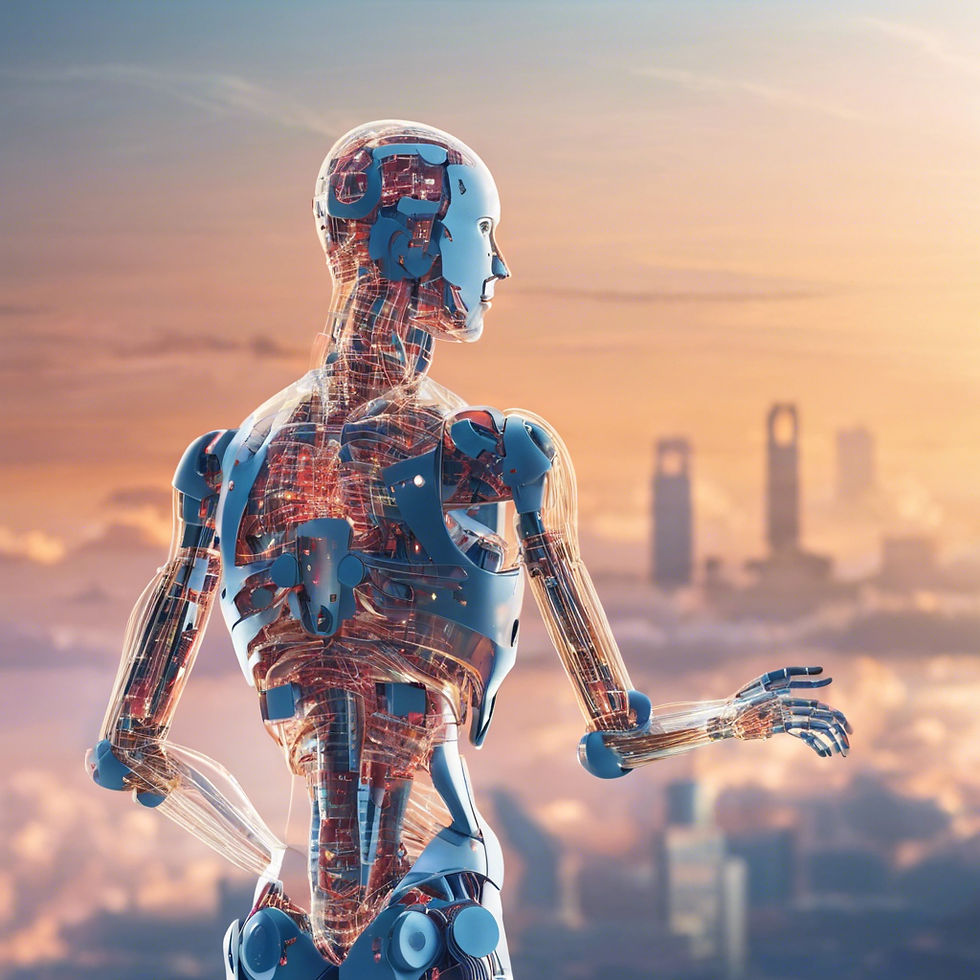
Additionally, policymakers must be cautious in interpreting the results of topic modeling, as the identified topics may not fully capture the complexity of public sentiment.
Transparency and accountability in the use of AI for policy analysis are essential, ensuring that methodologies are clear and that decisions made based on AI-generated insights are justifiable.
Conclusion
AI-driven topic modeling represents a transformative approach to identifying and prioritizing policy issues based on public discourse.
By leveraging advanced algorithms like LDA and NMF, policymakers can gain valuable insights into public sentiment and emerging trends.
However, it is essential to approach this powerful tool with careful consideration of ethical implications and the potential for bias.As AI continues to evolve, its role in shaping public policy will likely expand, offering new opportunities for informed decision-making and enhanced civic engagement.
The integration of AI in policy analysis not only empowers policymakers but also fosters a more responsive and accountable governance framework.
Commentaires