Big Data and Voter Behavior-Predictive Modeling Using Machine Learning
- Prof.Serban Gabriel
- Oct 13, 2024
- 3 min read
In the era where information is not just power but currency, understanding the intricacies of voter behavior through the lens of big data and machine learning has become paramount. The traditional paradigms of political polling have shown their limitations, especially in closely contested elections where conventional wisdom often fails.
This shift towards predictive modeling using machine learning algorithms represents a fusion of technology and political science, promising a more nuanced understanding of voter preferences and behavior.
Big data in politics encompasses a multitude of data points, ranging from social media sentiment on platforms like X to consumer behavior data, and voter registration files.
The advent of machine learning has allowed for the processing of these vast datasets with tools like decision trees, neural networks, and ensemble methods.
These algorithms, unlike human analysts, can detect patterns and trends at scale, offering predictive insights into voter behavior that were previously unattainable.
Historically, political campaigns relied heavily on polls, which are snapshots of public opinion at a given moment.
However, with the rise of predictive analytics, as evidenced by successful models such as those used by YouGov, we see a pivot towards continuous, real-time analysis.
These models, learning from vast historical datasets, have demonstrated an ability to predict outcomes where polls have been notably off the mark.
The journey of predictive models in elections begins with extensive data collection.
This includes not just the structured data from voter registries but also the unstructured data from social media posts, news articles, and more.
Data pre-processing is crucial, involving cleaning, handling missing values, and data normalization to prepare it for analysis.
Feature engineering is a critical step where domain knowledge meets data science.
Here, variables influencing voting behavior are isolated, including demographic information, previous voting records, and real-time social media sentiment.
This data then feeds into machine learning models, which might range from simple logistic regression for binary outcomes to complex neural networks for capturing nuanced trends.
Model validation uses techniques like cross-validation or back-testing with known election results to ensure reliability.
However, the true test comes in real-time applications where these models must adapt to new incoming data continuously
A Scenario: Predictive Modeling in a Hypothetical Election
Consider a national election where data scientists employ predictive modeling to assess the chances of two major candidates.
They ingest social media reactions, economic indicators, and voter turnout forecasts.
Social Media Data: NLP is used to gauge sentiment towards candidates, revealing last-minute shifts in public opinion.
Economic Indicators: Real-time economic data influences models that predict which candidate might benefit from economic dissatisfaction or growth.
Voter Turnout: Historical turnout data, adjusted for current trends, predicts which demographics are likely to vote, thus swaying outcomes.
In this scenario, a model might predict a sudden uptick in support for one candidate due to a scandal involving the other, captured through an analysis of X posts and other social media reactions.
Ethical Considerations and Challenges
The use of big data in elections brings forth ethical dilemmas. Data biases can skew models to favor particular demographics or viewpoints.
Privacy issues arise as personal data becomes a tool for political campaigns.
There's also a call for transparency; the public deserves to understand how these models work and how they influence political decision-making.
Future Directions
The integration of real-time analytics could refine predictions right up to election day, making them more dynamic.
Cross-demographic and cross-national studies could explore how predictive models apply in different political climates, offering universal insights into voter behavior.
Conclusion
Predictive modeling in understanding voter behavior marks a significant evolution in political science, blending the art of politics with the science of data.
Yet, it demands a vigilant approach to ethics, ensuring that this technological leap does not come at the cost of democratic values.
As we move forward, the balance between leveraging technology for insight and maintaining the integrity of democratic processes will define the future of elections in the information age.
Mastering Political Marketing: Winning Strategies for Electoral Success: florin, serban gabriel: 9798334992085: Amazon.com: Books
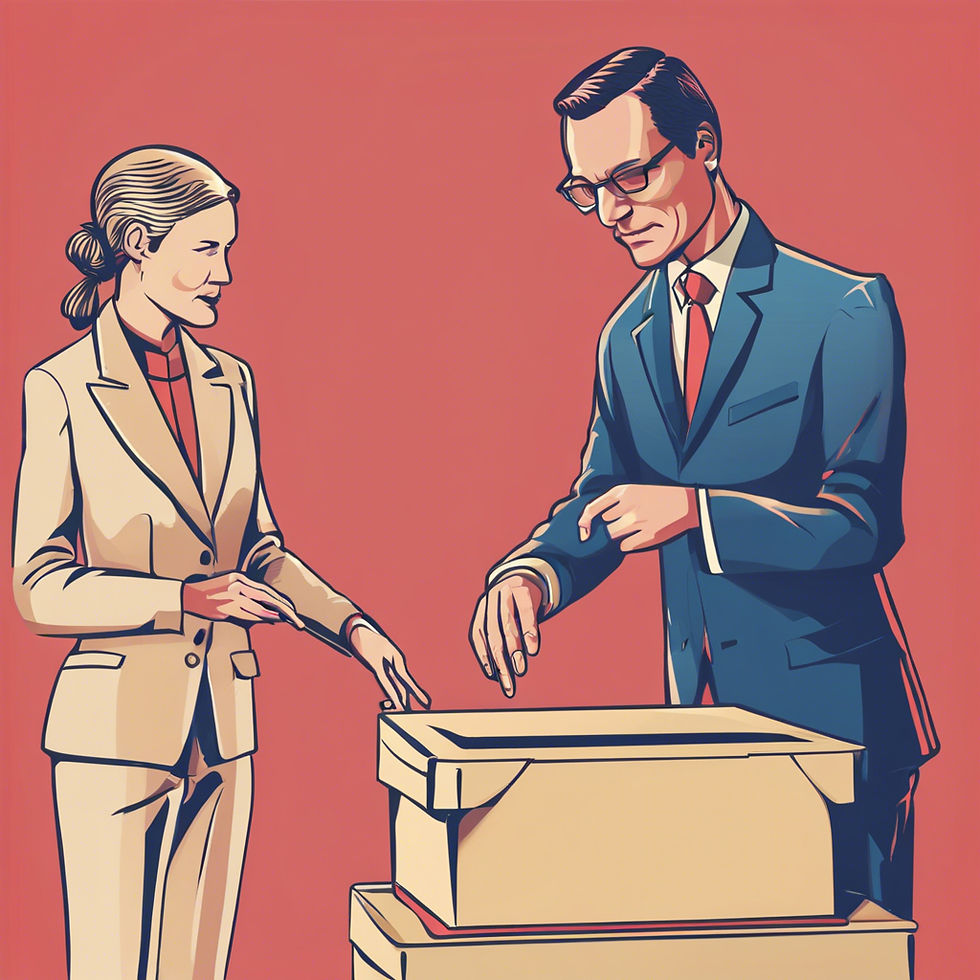
Bình luận