Can Machine Learning Decode the Unknown Patterns of Voter Behavior?
- Prof.Serban Gabriel
- Aug 16, 2024
- 4 min read
In the world of modern technology and data science, the realm of predicting voter behavior has been revolutionized by the application of machine learning algorithms. With the prowess of artificial intelligence and the vast expanse of data at our disposal, can we now unravel the enigmatic patterns of voter behavior that have mystified even the most seasoned political analysts?
Unveiling the Secrets of Voter Behavior Prediction
A New Frontier
Machine learning, a subset of artificial intelligence, has emerged as a powerful tool in decoding seemingly unpredictable human behavior.
By meticulously analyzing massive datasets spanning demographics, socio-economic factors, historical voting records, and more, machine learning algorithms can uncover hidden correlations and patterns that were previously imperceptible to the human eye.
Predictive analytics algorithms are used to forecast voter behavior and election outcomes based on historical data.
By analyzing past voting patterns, demographics, and socio-economic factors, campaigns can identify which segments of the population are most likely to support them.
Logistic Regression: This statistical method is commonly employed for binary classification tasks, such as predicting whether a voter will support a candidate or not. It estimates the probability of an event occurring based on one or more predictor variables.
Decision Trees: These algorithms create a model that predicts the value of a target variable based on several input features. Decision trees are particularly useful for understanding the factors that influence voter decisions, as they provide a clear visual representation of the decision-making process.
Voter segmentation algorithms allow campaigns to categorize voters into distinct groups based on shared characteristics.
This segmentation enables targeted messaging and outreach, increasing the effectiveness of campaign strategies.
K-Means Clustering: This unsupervised learning algorithm partitions voters into clusters based on similarities in their attributes (e.g., age, income, voting history). By identifying these clusters, campaigns can tailor their messaging to resonate with specific voter segments.
Hierarchical Clustering: Similar to K-means, hierarchical clustering organizes voters into a tree-like structure based on their similarities. This method can help campaigns understand the relationships between different voter groups and develop strategies accordingly.
NLP algorithms analyze text data from various sources, including social media, news articles, and public comments.
These algorithms help campaigns gauge public sentiment and understand voter opinions on key issues.
Sentiment Analysis: This technique uses NLP to determine the sentiment expressed in text data (positive, negative, or neutral). Campaigns can use sentiment analysis to monitor public opinion about candidates and issues, allowing them to adjust their messaging in real-time.
Topic Modeling: Topic modeling algorithms identify the main themes or topics within a corpus of text. By understanding the issues that are most relevant to voters, campaigns can tailor their communication strategies to address these topics.
The Power of Predictive Modeling
Through sophisticated predictive modeling techniques, machine learning can forecast voter sentiments, preferences, and even election outcomes with remarkable accuracy. By feeding algorithms with a plethora of relevant data points, these models can identify trends, detect anomalies, and make insightful predictions that transcend conventional analytical methods.
Harnessing Data for Insight
One of the key strengths of machine learning lies in its ability to sift through vast amounts of data efficiently. By employing algorithms like decision trees, support vector machines, or neural networks, researchers can distill complex datasets into actionable insights, shedding light on the underlying factors that drive voter behavior.
Challenges and Opportunities
While machine learning offers unprecedented capabilities in predicting voter behavior, it is not without its challenges. Ensuring data accuracy, avoiding biases, and interpreting model outputs correctly are crucial considerations in harnessing the full potential of these algorithms. However, by addressing these challenges proactively, researchers can unlock a wealth of opportunities in understanding and forecasting voter behavior.
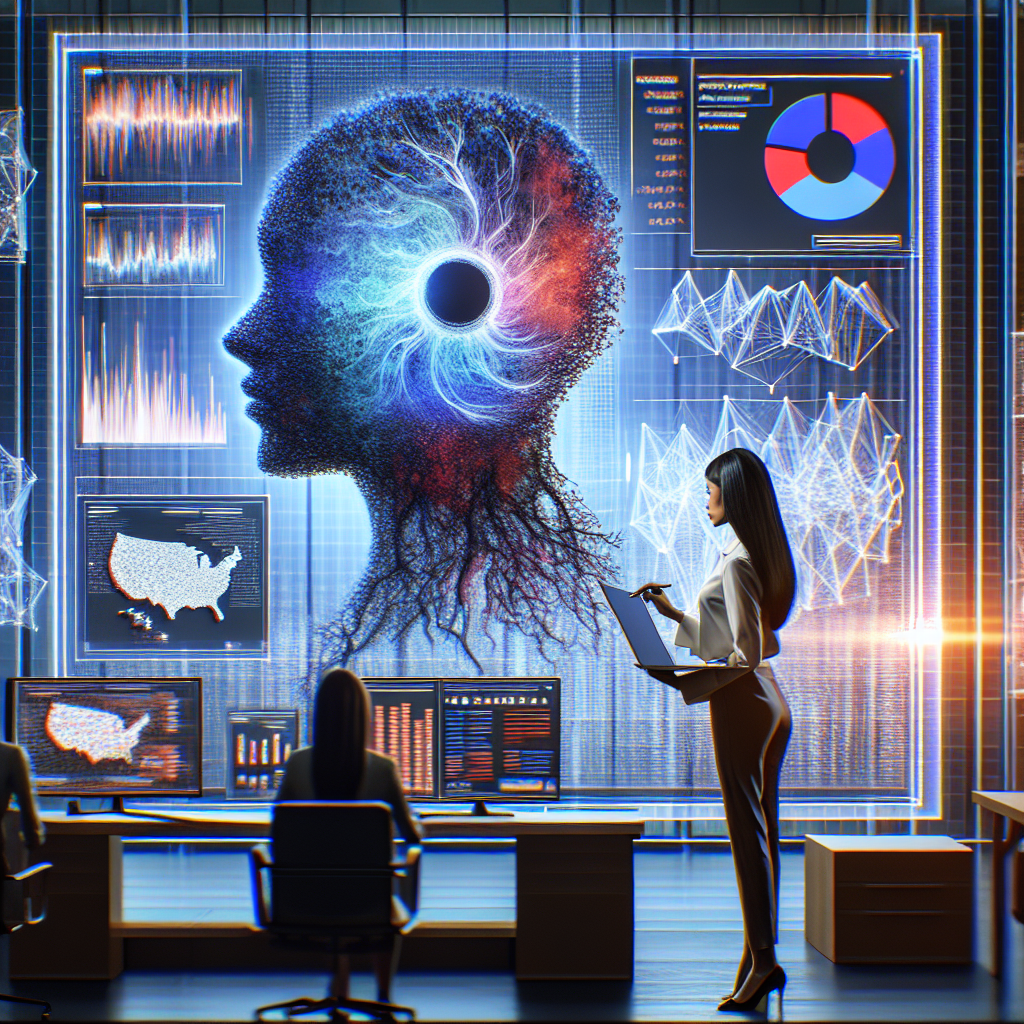
Looking Ahead: The Future of Voter Behavior Prediction
Ethical Considerations
As we delve deeper into the realm of machine learning-driven voter behavior predictions, ethical considerations loom large. Safeguarding data privacy, ensuring algorithmic transparency, and fostering trust in these predictive models are paramount in maintaining the integrity and fairness of the electoral process.
Democratizing Insights
The democratization of machine learning tools and techniques holds the promise of empowering a broader spectrum of researchers, analysts, and policymakers to delve into voter behavior prediction. By making these technologies more accessible and user-friendly, we can foster a more informed and data-driven approach to understanding electoral dynamics.
Continuous Evolution
The field of machine learning is in a state of perpetual evolution, with new algorithms, methodologies, and applications being developed at a rapid pace. Leveraging these innovations in the context of voter behavior prediction can open up new avenues for research, analysis, and decision-making in the political arena.
Conclusion
In our quest to decode the unknown patterns of voter behavior, machine learning stands out as a beacon of innovation and insight. By harnessing the power of data, algorithms, and predictive modeling, researchers are poised to unravel the intricacies of voter preferences and trends like never before. As we venture further into this data-driven landscape, let us tread carefully, ensuring that our pursuit of knowledge is guided by integrity, transparency, and a deep respect for the democratic process.
Let the algorithms unravel the mysteries, but let our ethics and values steer the way forward.
Stay tuned for more insights on the intersection of technology and politics!
Intrigued by the intersection of machine learning and voter behavior? Dive into the captivating world of data-driven insights with our future blog posts!
SEO Keywords: Voter Behavior Predictions, Machine Learning, Predictive Modeling, Data Science, Artificial Intelligence, Ethical Considerations
Comments