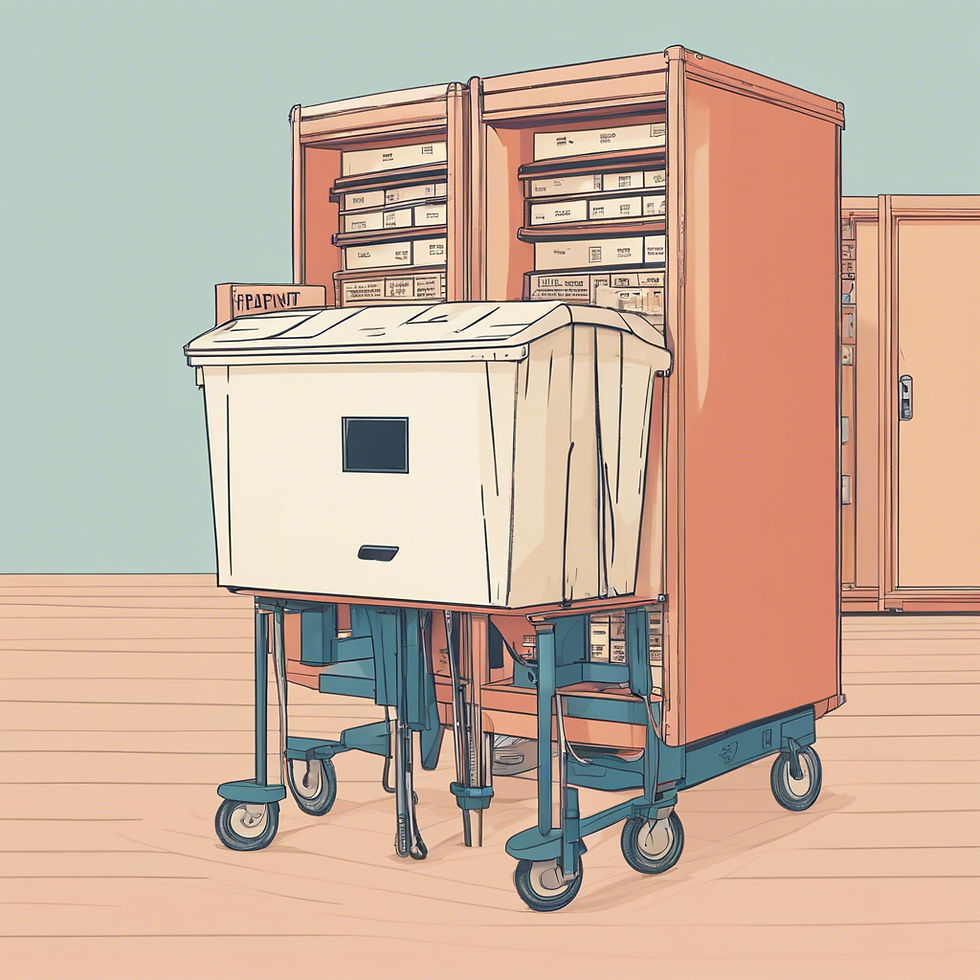
In an era where data is increasingly pivotal to decision-making processes, the protection of individual privacy rights has become a paramount concern, especially in sensitive areas such as voter databases.
Differential privacy (DP) emerges as a robust framework designed to safeguard individual information while allowing for meaningful data analysis.
This comprehensive exploration delves into the mechanisms of differential privacy, its implications for voter databases, and the broader context of individual rights in the data age.
Understanding Differential Privacy
Differential privacy is a mathematical framework that provides a quantifiable measure of privacy protection when analyzing datasets.
The core principle is that the inclusion or exclusion of a single individual's data should not significantly affect the outcome of any analysis.
This is achieved by introducing controlled noise into the dataset, thereby obscuring individual contributions while still allowing for aggregate insights.
The formal definition states that a mechanism is considered differentially private if, for any two datasets that differ by one record, the probability of any outcome produced by the mechanism is nearly identical.
This ensures that an observer cannot infer whether a particular individual's data was included in the analysis, regardless of their knowledge about the dataset or the analysis process itself
Mechanisms of Differential Privacy
Several mechanisms are employed to achieve differential privacy:
Laplacian Mechanism: Adds noise drawn from a Laplace distribution to query results based on sensitivity—a measure of how much a single individual's data can influence the output.
Gaussian Mechanism: Similar to the Laplacian mechanism but uses Gaussian noise, which can be more suitable in certain contexts where higher accuracy is needed.
Randomized Response: A technique where respondents answer sensitive questions with a probability that protects their true respons
SHADOWS OF POWER-Challenging Authority-A Critical Examination of Power Beyond the State: Florin, Serban Gabriel: 9798876049018: Amazon.com: Books
Anarchism Reloaded by Serban Gabriel, Paperback | Barnes & Noble® (barnesandnoble.com)
This method has been successfully applied in voting systems to maintain voter anonymity while collecting aggregate data
Differential Privacy in Voter Databases
The application of differential privacy in voter databases holds significant promise for protecting individual rights while enabling robust electoral analysis.
By implementing DP, electoral bodies can ensure that sensitive information about voters—such as their political affiliations or voting behaviors—remains confidential even as aggregate statistics are derived from this data.
Benefits of Differential Privacy in Voting
Enhanced Privacy: Voters can participate without fear of their choices being traced back to them, thus fostering greater participation and trust in the electoral process.
Data Utility: While DP introduces noise, it allows for valuable insights into voting patterns and demographics without compromising individual identities.
Regulatory Compliance: With stringent regulations like GDPR and CCPA emphasizing data protection, differential privacy provides a compliant framework for handling personal information
Challenges and Considerations
Despite its advantages, implementing differential privacy in voter databases presents challenges:
Balancing Privacy and Accuracy: The introduction of noise can lead to less accurate results, which may impact policy decisions based on electoral data. Striking a balance between privacy guarantees and data utility is crucial
Public Perception and Trust: Voter education about how differential privacy works is essential to build trust in its implementation. Misunderstandings about data anonymization techniques could lead to skepticism regarding election integrity.
Technical Complexity: Implementing differential privacy requires sophisticated algorithms and infrastructure, which may pose challenges for smaller electoral bodies with limited resources
Case Studies and Applications
Recent implementations of differential privacy highlight its effectiveness in protecting voter information:
The U.S. Census Bureau's adoption of differential privacy methods during the 2020 Census aimed to prevent re-identification of individuals while maintaining the utility of census data for public policy decisions.
This approach has sparked debates regarding its impact on social science research and policy formulation due to potential inaccuracies introduced by noise
Local differential privacy mechanisms have been tested in voting systems, demonstrating that they can effectively aggregate votes while preserving individual anonymity.
Studies show that these methods can maintain election integrity even with large populations
Conclusion
Differential privacy represents a significant advancement in protecting individual rights within voter databases amidst growing concerns over data privacy.
By employing robust mathematical frameworks that ensure anonymity while allowing for meaningful analysis, electoral bodies can enhance public trust and participation in democratic processes.
As technology evolves, ongoing research and development will be crucial in refining these methods to address emerging challenges and ensure that individual rights are upheld in an increasingly data-driven world.In summary, while differential privacy offers promising solutions for safeguarding voter information, careful consideration must be given to its implementation to balance privacy with the need for accurate and useful electoral data.
Comments