Mastering Political Marketing: Winning Strategies for Electoral Success
- Prof.Serban Gabriel
- Dec 23, 2024
- 4 min read
In today's world, where competition and media influence reign supreme, understanding political marketing is crucial for any aspiring leader or party.
Throughout this book, we will explore the intricacies of modern political tactics, revealing effective methods to gain public support and shape opinions in our rapidly evolving digital age.
As the author of this book, I've drawn inspiration from my earlier works, "Anarchism Reloaded" and "Shadows of Power," to offer you a fresh perspective on contemporary politics.
Proud to present groundbreaking theories in political analysis, aiming to enrich your understanding and empower you to navigate the complexities of today's political landscape.
With a captivating style and deep analysis, "Mastering Political Marketing: Winning Strategies" not only uncovers the secrets behind successful political campaigns but also provides practical strategies to turn your political vision into reality.
Whether you're a candidate looking for innovative election strategies or a political strategist eager to decode media dynamics, this book is your indispensable guide to mastering the art of political marketing in the 21st century.
Unlike traditional programming, where explicit instructions are given for every task, machine learning enables systems to find patterns and improve their performance over time through experience.
This capability has made machine learning a transformative force across various industries, including politics, healthcare, finance, and technology.
This essay explores the three main categories of machine learning - supervised learning, unsupervised learning, and reinforcement learning - and their applications in the political sphere.
Supervised Learning
In supervised learning, models are trained on labeled datasets, where the input data is paired with the correct output.
The goal is to learn a mapping from inputs to outputs, enabling the model to make predictions on new, unseen data.
Applications: Common applications include email filtering (classifying emails as spam or not), image recognition, and predictive analytics in political campaigns.
Example: Logistic regression, as discussed earlier, is a supervised learning algorithm used for binary classification tasks.
Supervised learning, which trains models on labeled datasets, has found significant use in political predictive analytics and voter segmentation.
By using historical data on voter demographics, campaign spending, and polling results, supervised learning models can predict election outcomes with increasing accuracy.
For instance, the accuracy of election predictions using machine learning has improved from approximately 60% in 2000 to 85% in 2020.
A prime example of supervised learning in political contexts is logistic regression, particularly effective for binary classification tasks such as predicting whether a candidate will win or lose.
The logistic regression model is defined by the equation:
P(Y=1|X) = 1 / (1 + e^ (- (β₀ + β₁X)))
Where P(Y=1|X) stands for the probability of the outcome given the predictor X, β₀ and β₁ are coefficients estimated from the data, and e is the base of the natural logarithm.
Unsupervised Learning
Unsupervised learning involves training models on unlabeled datasets, where the algorithm must find patterns and relationships within the data without predefined categories.
Applications: This type of learning is used in clustering (grouping similar items), anomaly detection (finding unusual data points), and market basket analysis (understanding consumer purchasing behavior).
Example: K-means clustering can be used to segment voters based on their preferences and behaviors.
Unsupervised learning, which shows patterns in unlabeled data, has proven valuable for clustering voters and topic modeling.
By grouping voters based on their preferences and behaviors, campaigns can better understand their electorate and tailor their messaging accordingly.
The use of sentiment analysis on social media in campaigns has seen a 300% increase between 2016 and 2020, highlighting the growing importance of unsupervised learning techniques.
K-means clustering, a popular unsupervised algorithm, has been effectively used to segment voters into groups with similar characteristics.
Campaigns using AI-based voter segmentation report an average 25% increase in messaging effectiveness.
Reinforcement Learning
Reinforcement learning is a type of machine learning where an agent learns to make decisions by interacting with an environment and receiving feedback in the form of rewards or penalties.
Applications: This approach is commonly used in robotics, game playing (e.g., AlphaGo), and perfecting campaign strategies based on real-time voter engagement.
Example: A political campaign could use reinforcement learning to adjust its outreach strategies based on voter responses and engagement metrics.
Reinforcement learning, where an agent learns through interaction with an environment, has found applications in improving dynamic campaign strategies and resource allocation.
This approach allows campaigns to adapt their strategies in real-time based on voter interactions and engagement.
Resource allocation models based on reinforcement learning have led to a 15-20% improvement in campaign spending efficiency.
This significant enhancement proves the potential of reinforcement learning in maximizing the impact of limited campaign resources.
Advanced Techniques and Evaluation Metrics
Beyond these core categories, political AI has also benefited from advanced techniques such as semi-supervised learning, transfer learning, and ensemble methods.
Transfer learning techniques, for instance, have reduced the time needed for adapting strategies between regions by up to 60%, while ensemble methods have improved voter turnout prediction accuracy by 10-15% compared to individual models.
To evaluate the performance of these models, political data scientists employ various metrics:
Entropy: H(X) = -Σ P(x) log₂ P(x)
Used to measure uncertainty in predictions.
Precision: True Positive / (True Positive + False Positive)
Evaluates the accuracy of positive predictions.
Recall: True Positive / (True Positive + False Negative)
Measures the model's ability to find all positive instances.
F1 Score: 2 (Precision Recall) / (Precision + Recall)
Provides a balanced measure of precision and recall.
Gini Coefficient: G = (2 Σ(i y_i) / (n * Σy_i)) - (n + 1) / n
Measures inequality in vote distribution.
Conclusion
The application of machine learning in political AI has revolutionized campaign strategies, voter outreach, and election predictions.
As these technologies continue to evolve, they promise even greater insights and efficiencies in political processes.
However, it's crucial to consider the ethical implications of these powerful tools and ensure their responsible use in keeping the integrity of democratic processes.
The future of political AI lies in the integration of these various machine learning techniques, creating more sophisticated and correct models that can navigate the complex landscape of modern politics.
As we move forward, the challenge will be to balance the power of these tools with transparency and fairness, ensuring that AI enhances rather than undermines the democratic process.
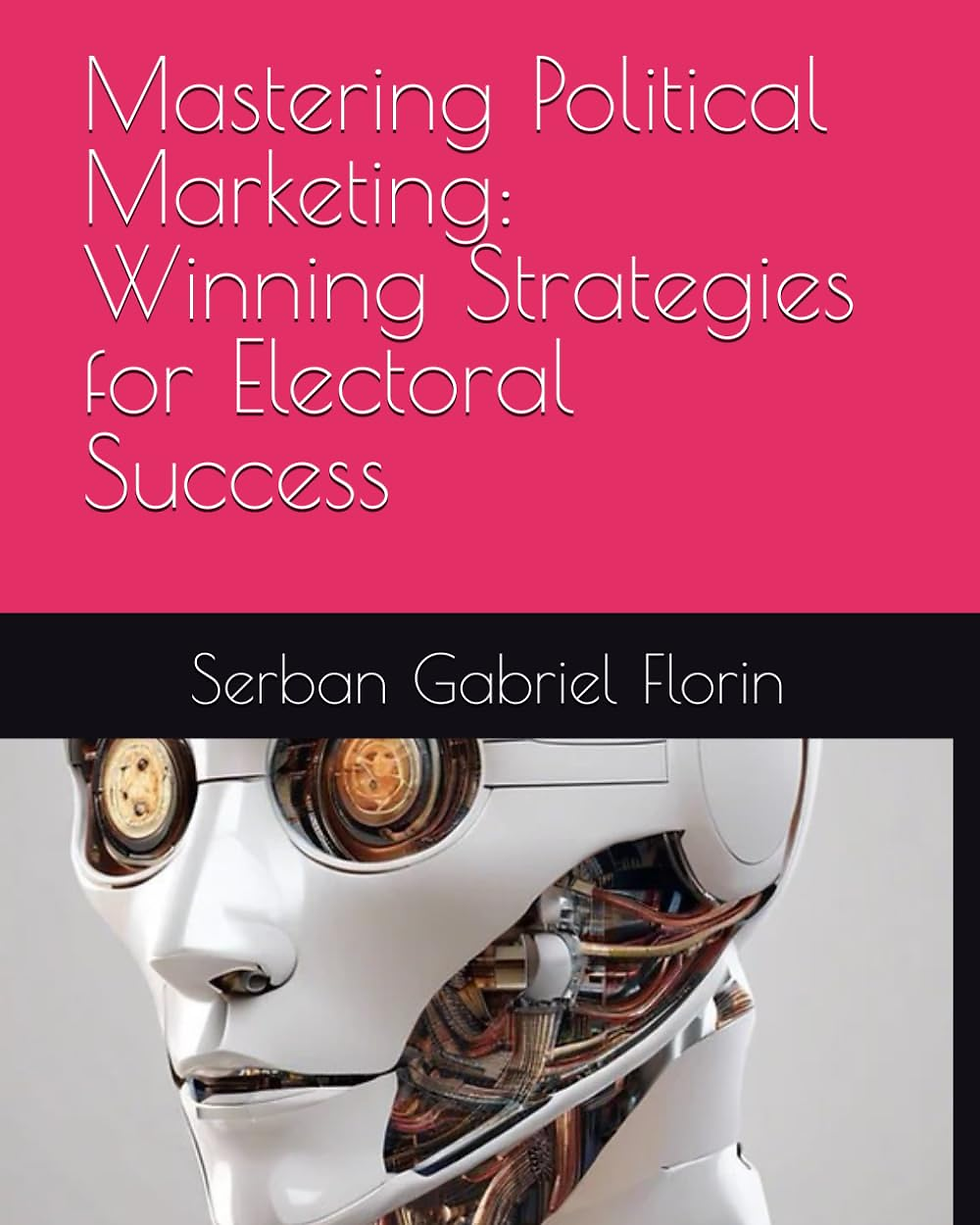
Comments