"Polls Plus: Supercharging Traditional Surveys with AI"
- Prof.Serban Gabriel
- Aug 23, 2024
- 3 min read
The integration of artificial intelligence (AI) into traditional polling methodologies is transforming the landscape of public opinion research.
This fusion, often termed "AI-enhanced polling," combines time-honored survey techniques with cutting-edge technologies to address longstanding challenges in the field while opening new avenues for insight.
At the core of this revolution are machine learning algorithms, natural language processing (NLP), and big data analytics.
These AI technologies are being deployed across various stages of the polling process, from sample selection to data analysis.
In sample selection, AI algorithms are tackling the persistent issue of non-response bias by analyzing vast datasets to identify patterns and correlations that might elude human researchers.
This allows for more nuanced and accurate weighting of survey results, ensuring better representation across demographic groups.
NLP is revolutionizing the analysis of open-ended survey responses.
Traditionally a labor-intensive and subjective process, NLP algorithms can now process thousands of responses rapidly, identifying themes, sentiment, and subtle linguistic nuances.
This not only increases efficiency but also allows for the inclusion of more open-ended questions, potentially capturing richer data.
In data collection, AI-powered chatbots and virtual interviewers are conducting surveys through text or voice interfaces.
These systems can adapt their questioning style based on respondents' answers and even detect emotional cues, leading to more engaging and informative interactions.
This dynamic approach has the potential to increase response rates and improve data quality.
A notable real-world application of AI-enhanced polling came from Morning Consult during the 2020 U.S. presidential election.
The firm employed a machine learning model to adjust for social desirability bias, addressing the phenomenon where respondents may be reluctant to express support for certain candidates due to perceived social pressures.
By analyzing patterns in response data and comparing them to actual voting behavior, the AI model identified and corrected for potential underreporting of support for certain candidates, leading to more accurate predictions.
However, the integration of AI into polling raises important epistemological and ontological questions.
Epistemologically, it challenges the traditional positivist assumption that public opinion can be objectively measured.
The complex nature of AI algorithms can make it difficult to scrutinize their reasoning, potentially leading to a false sense of certainty in research findings.
Ontologically, AI-enhanced polling suggests a more fluid conception of public opinion.
As AI systems interact with respondents in real-time, potentially shaping the course of the survey, we must consider whether we are measuring pre-existing opinions or co-creating them through the survey process itself.
Ethical challenges also abound. Privacy concerns are paramount, as the combination of survey data with other sources of personal information raises questions about data protection and informed consent.
The potential for AI systems to manipulate or unduly influence respondents through personalized questioning techniques presents additional ethical dilemmas.
Furthermore, the "black box" nature of many machine learning algorithms can undermine transparency and public trust in polling results.
Despite these challenges, the future of AI-enhanced polling looks promising.
Predictive modeling based on AI-enhanced survey data could provide more accurate forecasts of election outcomes, consumer behavior, or social trends.
The integration of biometric data from wearable devices with survey responses could offer new insights into the physiological correlates of opinions and attitudes.
Virtual reality environments could create immersive survey experiences that capture more nuanced reactions to complex scenarios.
To illustrate the potential of AI-enhanced polling, consider a hypothetical scenario for predicting voter turnout.
A logistic regression model enhanced by machine learning could be represented as:
logit(P(T)) = β₀ + β₁S + β₂M + β₃H + f(X)
Where P(T) is the probability of an individual turning out to vote,
S represents survey responses,
M is a social media engagement score,
H is the historical voting record, and f(X) is a function learned by a machine learning algorithm that considers additional features X (such as demographic information and current events).
This model combines traditional survey data with social media analysis and historical records, continuously updating based on new data to provide real-time predictions.
As we navigate this evolving landscape, it is crucial to approach AI-enhanced polling with both enthusiasm for its potential and a critical eye towards its limitations and implications. By doing so, we can harness the power of AI to enhance our understanding of public opinion while maintaining the rigor and ethical standards essential to social science research.
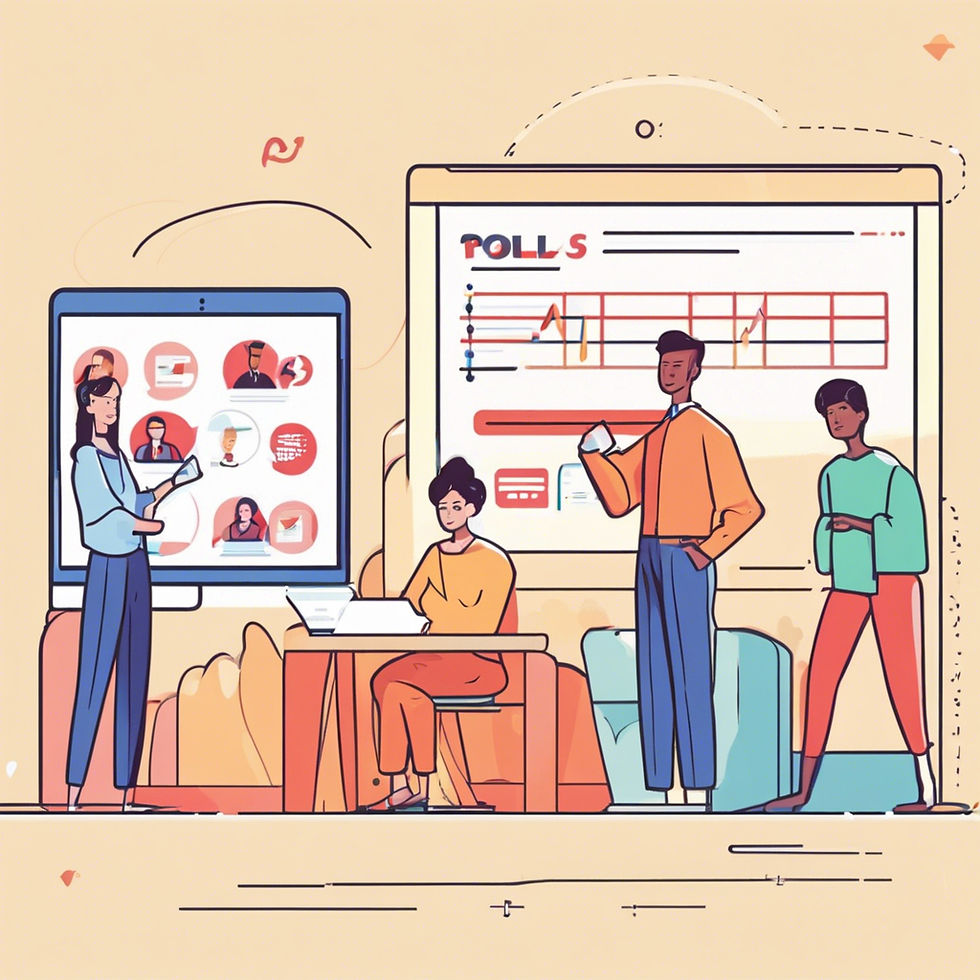
The future of polling lies in this delicate balance between technological innovation and methodological integrity, promising a new era of more accurate, nuanced, and comprehensive insights into societal attitudes and behaviors.
Comentarios