In the ever-evolving landscape of political campaigns, a new force has emerged that is reshaping the way candidates connect with voters: Artificial Intelligence (AI) micro-targeting. This sophisticated approach to voter outreach combines the power of big data analytics with machine learning algorithms to create highly personalized political messages.
In this blog post, we'll delve into the intricacies of AI micro-targeting, explore its impact on modern politics, and examine both its potential benefits and ethical concerns.
Understanding AI Micro-targeting
Definition and Context
AI micro-targeting in politics refers to the use of artificial intelligence and machine learning algorithms to analyze vast amounts of voter data and create highly personalized political messages tailored to specific individuals or small groups of voters.
This technique goes beyond traditional demographic segmentation, incorporating behavioral data, social media activity, and even psychographic profiles to predict voter preferences and behaviors with unprecedented accuracy.
Dr. Jennifer Stromer-Galley, Professor of Information Studies at Syracuse University, explains:
"AI micro-targeting represents a paradigm shift in political campaigning. It allows campaigns to move beyond broad-brush approaches and instead craft messages that resonate on an almost individual level. This technology has the potential to dramatically increase the efficiency and effectiveness of political outreach."
Key Components of AI Micro-targeting
Data Collection: The foundation of AI micro-targeting is vast amounts of data. This includes traditional voter registration information, but also extends to online behavior, social media activity, purchase history, and more.
Data Integration: AI systems combine disparate data sources to create comprehensive voter profiles.
Machine Learning Algorithms: These algorithms analyze the integrated data to identify patterns and make predictions about voter behavior and preferences.
Message Customization: Based on the AI's analysis, political messages are tailored to appeal to specific individuals or small groups.
Multi-channel Delivery: Personalized messages are delivered through various channels, including social media, email, direct mail, and even door-to-door canvassing.
The Evolution of Political Targeting
To fully appreciate the impact of AI micro-targeting, it's essential to understand its historical context.
From Mass Media to Micro-targeting
Mass Media Era (1950s-1990s): Political campaigns relied heavily on broadcast television and radio to reach broad audiences with general messages.
Early Digital Era (1990s-2000s): The rise of the internet and email allowed for more targeted communication, but still largely based on broad demographic categories.
Social Media Era (2000s-2010s): Platforms like Facebook and Twitter enabled more precise targeting based on interests and online behavior.
AI Micro-targeting Era (2010s-Present): The integration of big data analytics and machine learning has ushered in an era of hyper-personalized political communication.
Dr. Eitan Hersh, Associate Professor of Political Science at Tufts University, notes:
"The progression from mass media to AI micro-targeting reflects a fundamental shift in how campaigns view voters. We've moved from seeing the electorate as large, monolithic blocs to understanding voters as individuals with unique concerns and motivations."
The Science Behind AI Micro-targeting
Data Science and Machine Learning
At its core, AI micro-targeting relies on advanced data science techniques and machine learning algorithms. These tools allow campaigns to process and analyze vast amounts of data to extract meaningful insights.
Key techniques include:
Predictive Modeling: Using historical data to forecast future behavior or preferences.
Clustering Algorithms: Grouping similar voters together based on multiple attributes.
Natural Language Processing (NLP): Analyzing text data from social media posts, emails, and other sources to understand voter sentiment and concerns.
Deep Learning: Using neural networks to identify complex patterns in voter behavior that might not be apparent through traditional analysis.
Dr. Michal Kosinski, Associate Professor of Organizational Behavior at Stanford Graduate School of Business, explains:
"The power of AI in micro-targeting comes from its ability to identify nuanced patterns in vast datasets. Traditional methods might tell you that a voter is a 35-year-old woman living in the suburbs.
AI can tell you that she's concerned about climate change, prefers policy-focused messages, and is most likely to engage with political content on Instagram between 8 and 9 PM."
Psychographics and Behavioral Analysis
One of the most powerful aspects of AI micro-targeting is its ability to incorporate psychographic and behavioral data into its analysis.
This goes beyond traditional demographics to understand the psychological characteristics that influence political behavior.
Key concepts include:
OCEAN Model: Also known as the Big Five personality traits (Openness, Conscientiousness, Extraversion, Agreeableness, and Neuroticism), this framework helps categorize individuals based on their psychological characteristics.
Behavioral Economics: Incorporating insights from fields like prospect theory to understand how voters make decisions under uncertainty.
Sentiment Analysis: Using NLP techniques to gauge the emotional tone of a voter's online communications.
Dr. Sandra González-Bailón, Associate Professor at the Annenberg School for Communication, University of Pennsylvania, notes:
"By incorporating psychographic data, AI micro-targeting can tap into the underlying motivations and values that drive political behavior. This allows campaigns to craft messages that resonate on an emotional level, potentially increasing their persuasive power."
The Art of Message Crafting
While the science of AI micro-targeting focuses on data analysis and prediction, the art lies in crafting compelling messages based on these insights.
Personalization at Scale
AI enables campaigns to create thousands of variations of their core message, each tailored to resonate with specific voter segments or even individuals. This might include:
Adjusting the tone and style of the message (e.g., formal vs. casual)
Emphasizing different policy points based on voter priorities
Using images and graphics that appeal to specific demographic or psychographic profiles
Dynamic Content Optimization
AI systems can continuously analyze the performance of different message variations and optimize in real-time. This allows campaigns to:
A/B test different messages at an unprecedented scale
Quickly identify and amplify the most effective content
Adapt messaging strategies as voter sentiments shift during the campaign
Dr. Philip N. Howard, Professor of Internet Studies at the Oxford Internet Institute, observes:
"The combination of AI-driven analysis and creative message crafting represents a new frontier in political communication. It allows campaigns to be both data-driven and emotionally resonant, a powerful combination in the quest to win hearts and minds."
A Hypothetical Scenario: The AI-Driven Campaign
To illustrate the potential impact of AI micro-targeting, let's consider a hypothetical scenario for a mayoral campaign in a mid-sized American city.
Scenario Setup
Candidate: Jane Smith, running for mayor of Metropolis (population 500,000) Campaign Goal: Increase voter turnout among 25-40 year old professionals and secure their support
The AI Micro-targeting Approach
Data Collection and Integration
Voter registration data
Social media activity (public posts, likes, shares)
Local news engagement
Census data
Consumer behavior data (anonymized and aggregated)
AI Analysis The campaign's AI system, which we'll call "CampaignAI," processes this data to create detailed voter profiles and identify key segments within the target demographic.
Voter Segmentation CampaignAI identifies several key segments within the 25-40 year old professional demographic: a) Tech-savvy environmentalists b) Young parents concerned about education c) Urban professionals focused on economic growth d) Health-conscious fitness enthusiasts
Message Customization For each segment, CampaignAI generates tailored messaging strategies: a) Tech-savvy environmentalists:
Emphasize Smith's plan for sustainable urban development
Highlight proposals for expanding public transportation and bike lanes
Use infographics showcasing potential reduction in carbon emissions
b) Young parents:
Focus on Smith's education reform plans
Share stories of local schools benefiting from pilot programs
Use images of diverse, happy families in campaign materials
c) Urban professionals:
Emphasize Smith's economic development initiatives
Share data on job growth potential in key industries
Use sleek, modern visuals in campaign materials
d) Health-conscious fitness enthusiasts:
Highlight Smith's plans for expanding public parks and recreation areas
Share her personal commitment to health and fitness
Use images of Smith participating in local sports events
Multi-channel Delivery CampaignAI determines the optimal channels and timing for reaching each segment: a) Tech-savvy environmentalists:
Primary channels: Twitter, LinkedIn
Best times: Weekday evenings, Sunday afternoons
b) Young parents:
Primary channels: Facebook, Instagram
Best times: Early mornings, after 8 PM
c) Urban professionals:
Primary channels: LinkedIn, Email
Best times: Lunch hours, Saturday mornings
d) Health-conscious fitness enthusiasts:
Primary channels: Instagram, fitness apps
Best times: Early mornings, weekend afternoons
Dynamic Optimization As the campaign progresses, CampaignAI continuously analyzes the performance of different message variations and adjusts the strategy accordingly.
Mathematical Approach: Engagement Scoring
To quantify the effectiveness of the micro-targeting strategy, CampaignAI uses an engagement scoring system.
For each voter in the target demographic, it calculates an Engagement Score (ES) based on their interactions with campaign content.
The Engagement Score is calculated as follows:
ES = (w1 C) + (w2 L) + (w3 S) + (w4 R)
Where: C = Number of clicks on campaign content L = Number of likes/reactions S = Number of shares R = Number of replies/comments w1, w2, w3, w4 = Weighting factors for each type of engagement
The weighting factors are determined by the AI based on their observed correlation with voter turnout and support in previous campaigns. For this scenario, let's assume the following weights:
w1 (clicks) = 0.2 w2 (likes) = 0.3 w3 (shares) = 0.4 w4 (replies) = 0.5
Example calculation for a single voter:
Voter Actions:
5 clicks on campaign content
3 likes
2 shares
1 reply
ES = (0.2 5) + (0.3 3) + (0.4 2) + (0.5 1) ES = 1 + 0.9 + 0.8 + 0.5 ES = 3.2
CampaignAI tracks the average Engagement Score for each voter segment and uses this data to refine its targeting and messaging strategies continually.
Results and Analysis
After implementing the AI micro-targeting strategy for one month, the campaign observes the following results:
Overall engagement among the target demographic increases by 45% compared to traditional targeting methods.
Voter segment breakdown: a) Tech-savvy environmentalists: 62% increase in engagement b) Young parents: 51% increase in engagement c) Urban professionals: 38% increase in engagement d) Health-conscious fitness enthusiasts: 29% increase in engagement
The campaign identifies a new, previously unrecognized voter segment: "Civic-minded remote workers," who show high engagement with content related to improving city infrastructure for digital connectivity.
Based on historical correlations between engagement scores and voting behavior, CampaignAI projects a 15% increase in voter turnout among the target demographic.
As AI technology continues to advance, we can expect even more sophisticated micro-targeting capabilities in future political campaigns. Some potential developments include:
Real-time Emotion Analysis: Using computer vision and NLP to analyze voters' emotional responses to campaign messages in real-time, allowing for immediate adjustments.
Predictive Voter Modeling: Creating detailed simulations of voter behavior to test campaign strategies before deployment.
AI-Generated Content: Using generative AI to create personalized videos, images, and text for each voter.
Cross-Platform Targeting: Developing more sophisticated methods for tracking and targeting voters across multiple digital platforms and devices.
The future of politics will undoubtedly be shaped by AI, but it's up to us to ensure that this future aligns with our values and strengthens, rather than undermines, our democratic institutions.
You can find complete details in my book.
Mastering Political Marketing: Winning Strategies for Electoral Success - Kindle edition by florin, serban. Politics & Social Sciences Kindle eBooks @ Amazon.com.
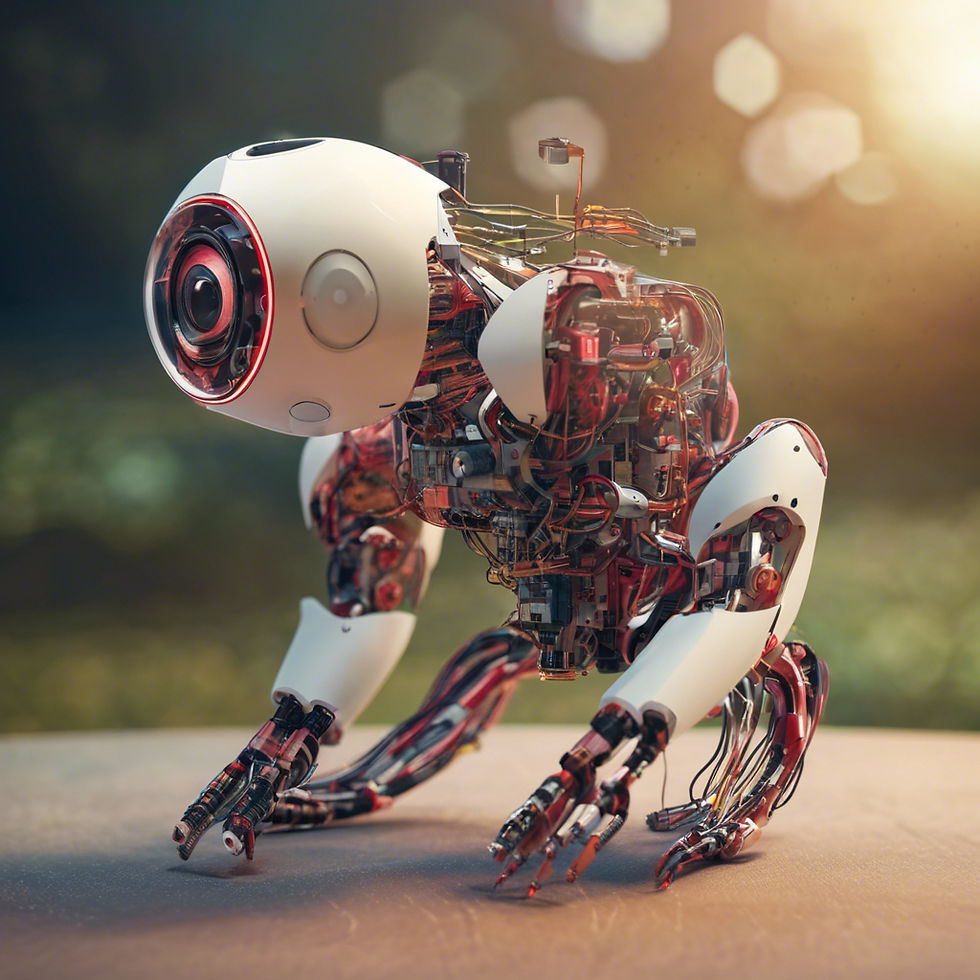
Opmerkingen