The Mind of the Voter: Merging Psychological Insights with AI Predictions"
- Prof.Serban Gabriel
- Aug 19, 2024
- 7 min read
Updated: Aug 21, 2024
Introduction
The intersection of artificial intelligence (AI) and political psychology has given rise to a new frontier in understanding and predicting voter behavior. As technology advances, political campaigns and researchers are increasingly turning to AI-powered tools to model complex psychological processes that influence voting decisions.
This essay explores the convergence of psychological insights and AI predictions in the realm of voter behavior, examining the potential benefits, challenges, and ethical considerations of this emerging field.
The use of AI in political campaigns has evolved from simple demographic targeting to sophisticated models that incorporate psychological principles, personality traits, and cognitive biases.
By leveraging machine learning algorithms and big data analytics, political strategists aim to create more accurate and nuanced predictions of voter behavior.
This approach not only promises to revolutionize campaign strategies but also raises important questions about the nature of democracy, free will, and the potential for manipulation of the electoral process.
Epistemological Concepts
Before delving into the specifics of AI-driven voter behavior modeling, it is essential to establish a framework for understanding how we acquire and validate knowledge in this domain.
Three key epistemological concepts are particularly relevant to this discussion:
Empiricism: The philosophical doctrine that emphasizes the role of sensory experience and evidence in the formation of ideas, over innate concepts or traditions. In the context of voter behavior prediction, empiricism underscores the importance of data-driven approaches and observable patterns.
Rationalism: The theory that reason is the chief source and test of knowledge, emphasizing the power of logical deduction and innate ideas. In AI-driven voter behavior modeling, rationalism is reflected in the development of theoretical frameworks and algorithms that attempt to capture the logical processes underlying decision-making.
Fallibilism: The epistemological view that human knowledge is inherently uncertain and subject to revision. This concept is crucial in understanding the limitations and potential errors in AI predictions of voter behavior, as well as the need for continuous refinement of models based on new data and insights.
These epistemological concepts provide a foundation for critically examining the methods and assumptions underlying the use of AI in predicting voter behavior.
Psychological Insights into Voter Behavior
To understand how AI is being used to model voter behavior, it is first necessary to examine the psychological principles that influence political decision-making.
Decades of research in political psychology have identified several key factors that shape voter preferences and actions:
Personality Traits: The "Big Five" personality traits (openness, conscientiousness, extraversion, agreeableness, and neuroticism) have been shown to correlate with political ideologies and voting patterns.
For example, openness to experience is often associated with more liberal political views, while conscientiousness tends to correlate with conservative leanings (Gerber et al., 2011).
Cognitive Biases: Various cognitive biases, such as confirmation bias, anchoring effect, and availability heuristic, influence how voters process political information and form opinions.
These biases can lead to selective exposure to information, resistance to changing views, and overestimation of the prevalence of one's own beliefs (Kahneman, 2011).
Emotional Responses: Emotions play a significant role in political decision-making, often overriding rational considerations.
Fear, anger, and enthusiasm have been shown to impact voter turnout and candidate preferences (Marcus et al., 2000).
Social Identity: Voters often align themselves with political parties or ideologies based on their social identities, such as race, religion, or socioeconomic status.
This phenomenon, known as social identity theory, can lead to in-group favoritism and out-group derogation in political contexts (Tajfel & Turner, 1979).
Moral Foundations: Research by Jonathan Haidt and colleagues suggests that individuals' moral intuitions, based on six fundamental moral foundations (care, fairness, liberty, loyalty, authority, and sanctity), strongly influence their political orientations and voting behavior (Haidt, 2012).
Information Processing Styles: Differences in how individuals process information, such as need for cognition, cognitive complexity, and tolerance for ambiguity, can impact their political attitudes and susceptibility to persuasion (Jost et al., 2003).
These psychological factors, among others, form the basis for understanding voter behavior and provide the foundation for AI-driven predictive models.
AI Techniques in Voter Behavior Prediction
Artificial intelligence, particularly machine learning and deep learning algorithms, offers powerful tools for analyzing and predicting voter behavior based on psychological insights. Some key AI techniques used in this domain include:
Natural Language Processing (NLP): NLP algorithms analyze text data from social media posts, news articles, and other online sources to gauge public opinion, sentiment, and political leanings.
These techniques can identify patterns in language use that correlate with psychological traits and political preferences.
Sentiment Analysis: AI-powered sentiment analysis tools assess the emotional tone of text and speech, helping to measure public mood and reactions to political events or candidates.
This information can be used to predict how emotions might influence voting decisions.
Social Network Analysis: Machine learning algorithms can map and analyze social networks to identify influential individuals, track the spread of political information, and predict how social connections might impact voting behavior.
Predictive Modeling: Advanced statistical techniques and machine learning algorithms, such as random forests, support vector machines, and neural networks, are used to build predictive models of voter behavior based on demographic, psychographic, and behavioral data.
Recommender Systems: Similar to those used in e-commerce, AI-powered recommender systems can suggest personalized political content to voters based on their psychological profiles and past behaviors.
Computer Vision: AI algorithms can analyze images and videos to detect emotional responses, assess crowd sizes at political events, and even analyze facial expressions during debates to gauge public reactions.
These AI techniques, combined with big data analytics, allow for the creation of highly sophisticated voter behavior models that incorporate psychological insights.
Scholarly Perspectives
Three prominent scholars have made significant contributions to the understanding of voter behavior and the application of AI in political contexts:
Cass Sunstein: A legal scholar and behavioral economist, Sunstein has explored the impact of information ecosystems on political polarization and decision-making. His work on "echo chambers" and "filter bubbles" is particularly relevant to understanding how AI-driven personalization of political content can influence voter behavior.
Sunstein argues that exposure to diverse viewpoints is essential for democratic deliberation and warns against the potential for AI to reinforce existing biases and polarization (Sunstein, 2017).
Michal Kosinski: A psychologist and data scientist, Kosinski has conducted groundbreaking research on the use of digital footprints to predict personality traits and political preferences.
His work demonstrates how AI can infer sensitive personal information from seemingly innocuous data, raising important questions about privacy and the ethical use of AI in political campaigns (Kosinski et al., 2013).
Diana Mutz: A political scientist specializing in political communication and public opinion, Mutz has studied the effects of media exposure on political attitudes and behavior.
Her research on cross-cutting political discourse and the impact of social networks on political tolerance provides valuable insights into how AI-driven targeting might affect democratic discourse and polarization (Mutz, 2006).
These scholars' work highlights both the potential and the risks associated with using AI to predict and influence voter behavior.
Applications and Implications
The integration of psychological insights with AI predictions has several important applications and implications for political campaigns and democratic processes:
Micro-targeting: AI-driven voter models allow campaigns to tailor their messages to specific individuals based on their psychological profiles, potentially increasing the effectiveness of political communication.
However, this raises concerns about manipulation and the erosion of shared political discourse.
Voter Turnout Prediction: By analyzing historical data and psychological factors, AI models can predict voter turnout with increasing accuracy, allowing campaigns to focus their resources more efficiently.
This could lead to more effective get-out-the-vote efforts but may also exacerbate existing inequalities in political participation.
Issue Framing: AI-powered analysis of psychological traits can help campaigns frame issues in ways that resonate with different voter segments.
While this could lead to more effective communication, it also raises questions about authenticity and the potential for exploiting psychological vulnerabilities.
Real-time Campaign Adjustment: AI models can process real-time data to help campaigns adjust their strategies quickly in response to changing public sentiment or unforeseen events.
This agility could make campaigns more responsive to voter concerns but may also lead to a focus on short-term reactions rather than long-term policy considerations.
Voter Persuasion: By leveraging psychological insights, AI-driven persuasion techniques could become increasingly sophisticated and effective.
This raises ethical concerns about the manipulation of voter preferences and the potential for eroding genuine political discourse.
Information Ecosystem Management: AI algorithms play a crucial role in shaping the information ecosystems that voters inhabit online.
The ability to personalize political content based on psychological profiles could lead to increased polarization and the reinforcement of existing beliefs.
Ethical Considerations and Challenges
The use of AI to model and predict voter behavior based on psychological principles raises several ethical concerns and challenges:
Privacy: The collection and use of personal data, including psychological profiles, for political purposes raises significant privacy concerns.
There is a need for clear regulations and transparency regarding data collection and usage in political campaigns.
Manipulation: The potential for AI-driven micro-targeting to exploit psychological vulnerabilities and manipulate voter behavior threatens the integrity of democratic processes.
Safeguards must be put in place to prevent undue influence and ensure that voters can make informed decisions.
Fairness and Equality: AI-powered campaign tools may exacerbate existing inequalities by allowing well-resourced campaigns to target voters more effectively. This could lead to certain groups being overlooked or underrepresented in political discourse.
Transparency and Accountability: The complexity of AI algorithms used in voter behavior prediction makes it difficult for the public to understand and scrutinize these tools. There is a need for greater transparency and accountability in the use of AI in political campaigns.
Echo Chambers and Polarization: AI-driven personalization of political content may reinforce existing beliefs and exacerbate political polarization, potentially undermining democratic deliberation and consensus-building.
Autonomy and Free Will: The increasing sophistication of AI-driven persuasion techniques raises philosophical questions about voter autonomy and the nature of free will in political decision-making.
Regulation and Governance: Developing appropriate regulations and governance frameworks for the use of AI in political campaigns is challenging due to the rapid pace of technological advancement and the complex ethical issues involved.
Conclusion
The convergence of psychological insights and AI predictions in modeling voter behavior represents a significant development in political campaigning and our understanding of democratic processes.
While this approach offers the potential for more effective and responsive political communication, it also raises important ethical concerns and challenges.
As AI technologies continue to evolve, it is crucial to strike a balance between leveraging these tools to enhance democratic engagement and protecting the integrity of the electoral process.
This will require ongoing dialogue between technologists, political scientists, ethicists, and policymakers to develop appropriate governance frameworks and ethical guidelines.
Furthermore, it is essential to promote digital literacy and critical thinking skills among voters to help them navigate an increasingly complex information landscape.
By fostering a more informed and discerning electorate, we can harness the potential of AI-driven voter behavior modeling while mitigating its risks.
Ultimately, the responsible development and deployment of AI in political contexts will play a crucial role in shaping the future of democracy in the digital age.
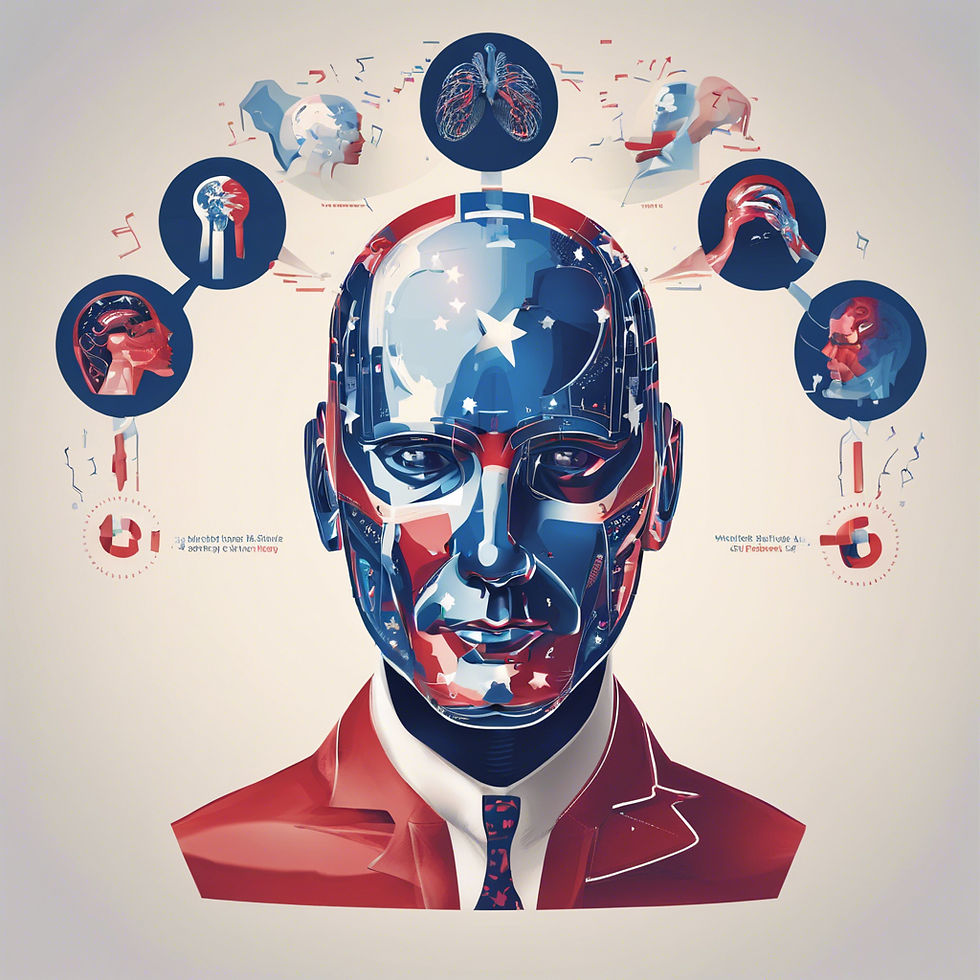
As we continue to explore this frontier, we must remain vigilant in upholding democratic values and ensuring that technological advancements serve to strengthen, rather than undermine, the foundations of representative government.
In my books, you will find comprehensive details on how to practically implement these themes.
Each topic is explored in depth, providing step-by-step guidance and actionable insights to help you apply the concepts effectively.
Whether you are looking for theoretical understanding or practical applications, my writings are designed to equip you with the knowledge and tools necessary for successful implementation.
Comentarios