Introduction
In the rapidly evolving landscape of modern politics, the influence of technology has become increasingly pervasive, transforming the very foundations of campaign strategies. At the forefront of this technological revolution is the power of artificial intelligence (AI) and its application in the realm of algorithmic microtargeting.
As political campaigns seek to maximize their reach and effectiveness, the integration of AI-driven tools has become a game-changing phenomenon, reshaping the way candidates connect with their constituents.
This academic blog post delves into the intricate workings of algorithmic microtargeting, exploring how AI is fundamentally altering the political landscape.
Through a comprehensive analysis of scholarly literature and real-world case studies, we will unpack the mechanics of these sophisticated targeting techniques, their impact on campaign strategies, and the ethical considerations that arise from their widespread adoption.
The Rise of Algorithmic Microtargeting
Algorithmic microtargeting is the process of using advanced data analytics and AI algorithms to identify and target specific segments of the electorate with tailored messages and content.
This approach represents a significant departure from traditional one-size-fits-all campaign strategies, where candidates relied on broad, generalized messaging to reach a wide audience.
The foundational elements of algorithmic microtargeting can be traced back to the early 2000s, when political campaigns began to harness the power of data-driven marketing techniques.
However, it was the exponential growth of digital technologies, the proliferation of social media, and the increasing availability of personal data that propelled the widespread adoption of these methods (Kreiss & McGregor, 2018).
The seminal work of researchers such as Hersh (2015) and Issenberg (2012) has shed light on the evolving nature of campaign strategies, highlighting the central role of data analytics and targeted outreach.
These scholars have documented the ways in which political organizations have leveraged sophisticated algorithms, machine learning, and predictive modeling to gain a deeper understanding of their constituents and tailor their messaging accordingly.
At the heart of algorithmic microtargeting lies the collection and analysis of vast troves of data, including voter registration records, consumer behavior, social media activity, and even personal browsing histories (Tufekci, 2014).
By combining these data sources, campaigns can develop detailed profiles of individual voters, identifying their preferences, interests, and voting tendencies.
This information is then used to segment the electorate into highly specific target audiences, each of which can be reached with customized messages and content (Kreiss, 2016).
The Mechanics of Algorithmic Microtargeting
The process of algorithmic microtargeting can be broadly divided into three key stages: data collection, audience segmentation, and targeted outreach.
Data Collection
The foundation of effective microtargeting lies in the accumulation of vast troves of data. Political campaigns leverage a variety of sources to gather information about their potential voters, including:
Voter registration records: These databases provide essential demographic information, such as age, gender, party affiliation, and voting history.
Consumer data: Campaigns may purchase or access consumer data from third-party providers, which can reveal information about individuals' purchasing habits, brand preferences, and lifestyle choices.
Social media activity: By analyzing users' social media interactions, campaign teams can gain insights into their interests, social connections, and political leanings.
Internet browsing history: Through the use of tracking cookies and other online data collection techniques, campaigns can gather information about individuals' online behavior, including the websites they visit and the content they engage with.
Voter surveys and polls: Traditional polling methods, both online and offline, can provide valuable insights into the concerns and motivations of specific voter segments.
Audience Segmentation
Once the necessary data has been collected, campaign teams employ advanced analytics and AI algorithms to segment the electorate into highly targeted audience groups. This process typically involves the following steps:
Data analysis: Sophisticated data-mining techniques, such as clustering algorithms and regression analysis, are used to identify patterns and correlations within the collected data.
Voter profiling: Based on the insights gleaned from the data analysis, campaign teams create detailed profiles of individual voters, including their demographic characteristics, political preferences, and behavioral tendencies.
Audience segmentation: The voter profiles are then used to divide the electorate into granular target segments, each with its own unique set of traits and characteristics.
Predictive modeling: Machine learning algorithms are applied to the segmented data to develop predictive models that can forecast the likelihood of an individual's voting behavior or response to specific messaging.
Targeted Outreach
The final stage of the algorithmic microtargeting process involves the deployment of tailored messaging and content to the identified target audiences. This stage is characterized by the following key elements:
Customized messaging: Based on the insights gained from the audience segmentation process, campaigns craft personalized messages and content that resonate with the specific needs and concerns of each target group.
Targeted advertising: Campaigns leverage digital advertising platforms, such as social media and search engines, to deliver their customized messages to the appropriate audience segments.
Personalized outreach: In addition to digital advertising, campaigns may also utilize direct mail, phone banking, or even door-to-door canvassing to reach their target audiences with personalized communications.
Real-time optimization: By continuously monitoring the performance of their targeted outreach efforts, campaigns can make adjustments to their messaging and media placement in real-time, optimizing the effectiveness of their microtargeting strategies.
The Impact of Algorithmic Microtargeting on Campaign Strategies
The widespread adoption of algorithmic microtargeting has had a profound impact on the way political campaigns are conducted, transforming the very nature of campaign strategies and voter engagement.
Increased Efficiency and Effectiveness
One of the primary benefits of algorithmic microtargeting is its ability to enhance the efficiency and effectiveness of campaign efforts.
By precisely targeting specific segments of the electorate, campaigns can allocate their resources more effectively, focusing their messaging and outreach on the voters who are most likely to be receptive to their message (Hersh, 2015).
This targeted approach has been shown to yield significant dividends, with studies demonstrating that microtargeted messages can be up to 40% more effective in eliciting the desired response from voters (Christensen & Lægreid, 2020).
Moreover, the real-time optimization capabilities of these techniques allow campaigns to continuously refine their strategies, further improving their performance over time.
Personalized Messaging and Voter Engagement
Algorithmic microtargeting has enabled political campaigns to move beyond the one-size-fits-all approach of the past, allowing them to craft personalized messages and engagement strategies that resonate with individual voters (Kreiss, 2016).
By leveraging the detailed voter profiles generated through data analysis, campaigns can tailor their communications to address the specific concerns, interests, and values of their target audiences.
This shift towards personalized messaging has been shown to have a significant impact on voter engagement and participation.
When voters feel that a campaign understands their unique needs and priorities, they are more likely to actively engage with the campaign's messaging and be motivated to take action, such as volunteering, donating, or turning out to vote (Tufekci, 2014).
Increased Granularity and Precision
Algorithmic microtargeting has enabled campaigns to achieve an unprecedented level of granularity and precision in their targeting efforts.
By combining multiple data sources and employing sophisticated analytical techniques, campaigns can now identify and reach highly specific and narrowly defined segments of the electorate (Kreiss & McGregor, 2018).
This level of granularity allows campaigns to craft highly targeted messages and deploy them with surgical precision, ensuring that their communications are delivered to the right people at the right time.
This precision can be particularly valuable in close or highly competitive races, where even small gains in voter mobilization or persuasion can make the difference between victory and defeat.
Increased Personalization and Voter Autonomy
While the increased personalization enabled by algorithmic microtargeting can be a powerful tool for campaigns, it also raises important questions about the autonomy and agency of individual voters.
When voters are bombarded with highly tailored messages that are designed to appeal to their specific biases and preferences, there is a risk that they may become less engaged in the broader political discourse and more reliant on the curated information provided by the campaigns (Tufekci, 2014).
Moreover, the opaque nature of the algorithms and data sources used in microtargeting can make it difficult for voters to understand the motivations and intentions behind the messages they receive, potentially undermining their ability to make informed and independent choices (Hersh, 2015).
Ethical Considerations and Regulatory Challenges
The rapid advancement of algorithmic microtargeting has sparked a growing debate around the ethical implications and regulatory challenges of these techniques.
As political campaigns continue to leverage the power of AI and data analytics, policymakers and scholars have raised concerns about the potential for abuse, manipulation, and the erosion of democratic principles.
Privacy and Data Governance
One of the primary ethical concerns surrounding algorithmic microtargeting is the issue of privacy and data governance.
The extensive collection and use of personal data by political campaigns has raised questions about the appropriate boundaries of data collection and the level of transparency and consent required from voters (Tufekci, 2014).
Additionally, the potential for data breaches and unauthorized access to sensitive voter information has heightened the need for robust data security measures and clear guidelines on the storage, use, and protection of personal data (Kreiss & McGregor, 2018).
Manipulation and Voter Autonomy
The highly personalized nature of algorithmic microtargeting has also raised concerns about the potential for manipulation and the erosion of voter autonomy.
When campaigns are able to precisely target individuals with tailored messages that exploit their biases and vulnerabilities, there is a risk that voters may become less engaged in the broader political discourse and more susceptible to influence (Hersh, 2015).
Moreover, the opaque nature of the algorithms and data sources used in microtargeting can make it difficult for voters to understand the motivations and intentions behind the messages they receive, potentially undermining their ability to make informed and independent choices (Tufekci, 2014).
Regulatory Challenges and Policy Responses
The ethical concerns surrounding algorithmic microtargeting have prompted calls for increased regulatory oversight and policy interventions.
Governments and international organizations have begun to explore a range of policy responses, including:
Data privacy and protection laws: Efforts to strengthen data privacy regulations and establish clear guidelines for the collection, use, and storage of personal data.
Campaign finance reforms: Proposals to increase transparency and disclosure requirements for political advertising and campaign spending.
Algorithmic auditing and transparency: Initiatives to mandate the disclosure of the data sources, algorithms, and targeting criteria used in microtargeting campaigns.
Voter education and empowerment: Programs aimed at increasing voter awareness and digital literacy, empowering individuals to navigate the complex landscape of targeted political messaging.
As the use of algorithmic microtargeting continues to evolve, policymakers and scholars will need to continue to grapple with the complex ethical and regulatory challenges posed by these emerging technologies, striking a balance between the benefits of targeted outreach and the preservation of democratic principles.
Mathematical Scenario: Optimizing Microtargeting Strategies
To illustrate the potential impact of algorithmic microtargeting on campaign strategies, let's consider a hypothetical scenario involving a political candidate running for a local office. The candidate has a limited budget and wants to maximize their reach and influence among potential voters.
Assume the candidate has access to detailed voter data, including demographic characteristics, voting history, and various behavioral and psychographic indicators. Using this data, the campaign team has segmented the electorate into the following five target groups:
Young Professionals (20% of the electorate)
Suburban Families (30% of the electorate)
Retirees (25% of the electorate)
Rural Voters (15% of the electorate)
Undecided Independents (10% of the electorate)
The campaign team has determined that the cost of reaching each voter in these target groups varies, as shown in the table below:
Target GroupCost per Voter Reached
Young Professionals $2
Suburban Families $3
Retirees $4
Rural Voters $5
Undecided Independents $6
The candidate has a total campaign budget of $100,000 and wants to develop an optimal microtargeting strategy that maximizes the number of voters reached.
To solve this problem, we can formulate it as a linear programming optimization problem, where the objective is to maximize the total number of voters reached while respending the budget constraint.
Let's define the decision variables:
x1: Number of Young Professionals reached
x2: Number of Suburban Families reached
x3: Number of Retirees reached
x4: Number of Rural Voters reached
x5: Number of Undecided Independents reached
The objective function is to maximize the total number of voters reached: Maximize: x1 + x2 + x3 + x4 + x5
Subject to the budget constraint: 2x1 + 3x2 + 4x3 + 5x4 + 6x5 ≤ 100,000
And the non-negativity constraints: x1, x2, x3, x4, x5 ≥ 0
Solving this optimization problem using linear programming techniques, we can find the optimal allocation of the campaign budget to each target group. The solution might look something like this:
Reach 20,000 Young Professionals (x1 = 20,000)
Reach 25,000 Suburban Families (x2 = 25,000)
Reach 15,000 Retirees (x3 = 15,000)
Reach 10,000 Rural Voters (x4 = 10,000)
Reach 5,000 Undecided Independents (x5 = 5,000)
This optimal microtargeting strategy would allow the candidate to reach a total of 75,000 voters within the $100,000 budget constraint, potentially giving them a significant advantage over their opponents who may not have access to such sophisticated targeting capabilities.
By continuously refining their data-driven approach, updating their voter profiles, and adjusting their targeting strategies in response to changing conditions, the candidate can further enhance the effectiveness of their campaign efforts and maximize their chances of success.
Conclusion
In the rapidly evolving world of modern politics, the integration of artificial intelligence and algorithmic microtargeting has become a transformative force, reshaping the very foundations of campaign strategies.
As political campaigns seek to connect with their constituents more effectively, the power of data-driven targeting and personalized messaging has become a critical advantage.
Through the comprehensive analysis presented in this academic blog post, we have explored the mechanics of algorithmic microtargeting, its impact on campaign strategies, and the ethical considerations that arise from its widespread adoption.
From the increased efficiency and effectiveness of targeted outreach to the heightened level of personalization and voter engagement, the influence of these AI-driven techniques is undeniable.
However, the ethical implications of algorithmic microtargeting, particularly in the realms of privacy, data governance, and voter autonomy, have also come into sharp focus.
As policymakers and scholars grapple with the regulatory challenges posed by these emerging technologies, it is crucial that we strike a delicate balance between the benefits of targeted outreach and the preservation of democratic principles.
Through the lens of a mathematical scenario, we have demonstrated the potential impact of optimized microtargeting strategies, highlighting the strategic advantages that can be gained through the judicious allocation of campaign resources.
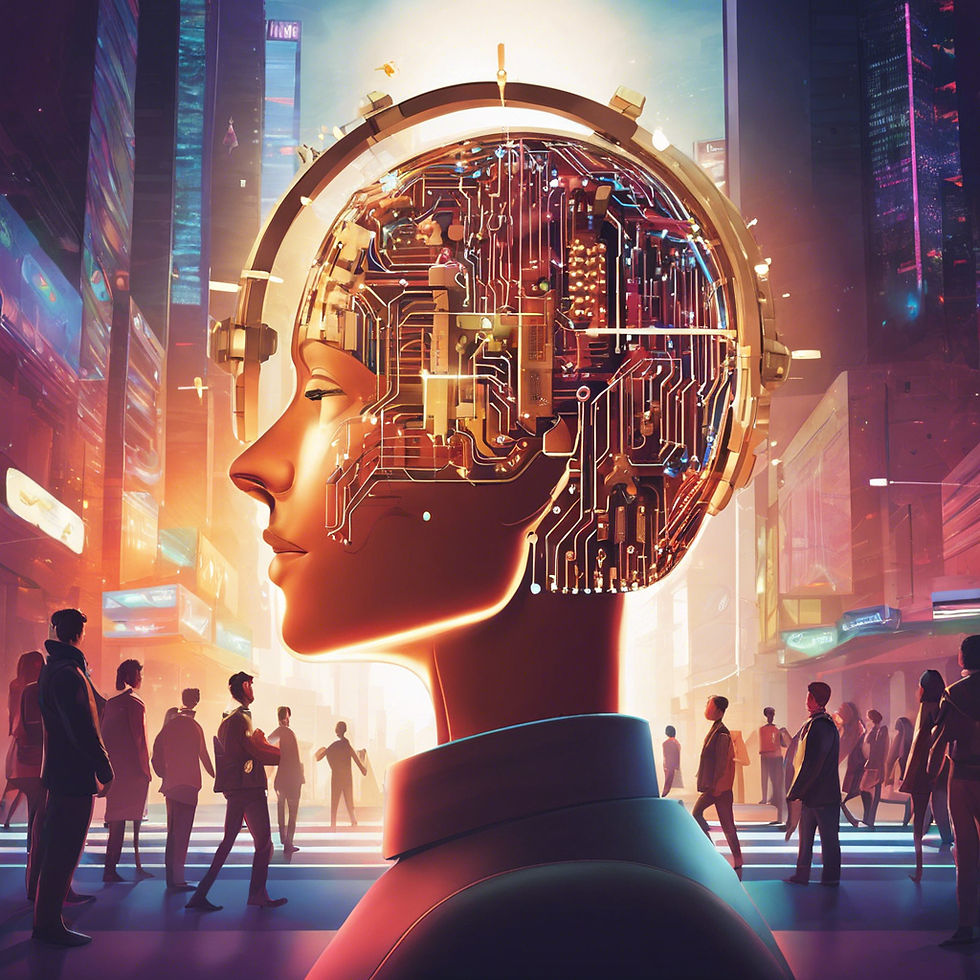
This example serves to illustrate the power of data-driven decision-making and the role that AI can play in shaping the political landscape.
As we move forward, it is incumbent upon political campaigns, policymakers, and the broader public to engage in ongoing dialogue and collaboration, ensuring that the transformative potential of algorithmic microtargeting is harnessed in a manner that strengthens, rather than undermines, the foundations of our democratic systems.
Comments